Approach
For the ith sample of class ci, we create twin augmentations (c̃ai, c̃bi), by sampling from a Gaussian centered at class embedding (µci). After this, we concatenate them with the same zi and obtain (w̃ai , w̃bi) from the mapping network, which we stack in batches of augmented latents (W̃A and W̃B). The twin (w̃ai, w̃bi) vectors are then made invariant to augmentations (similar) in the latent space by minimizing cross-correlation between the latents of two augmented batches (W̃A and W̃B).
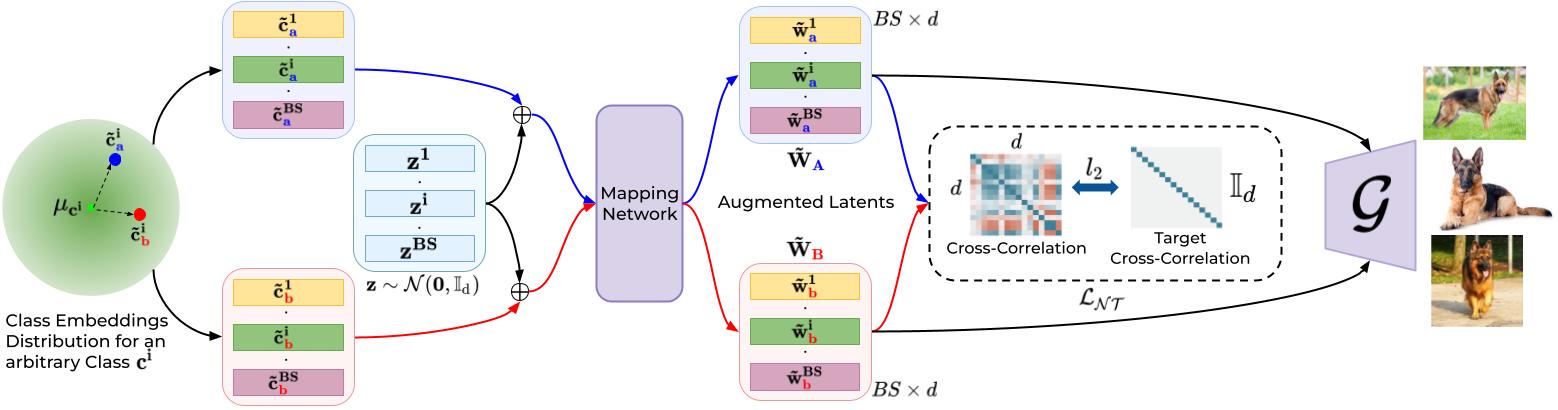